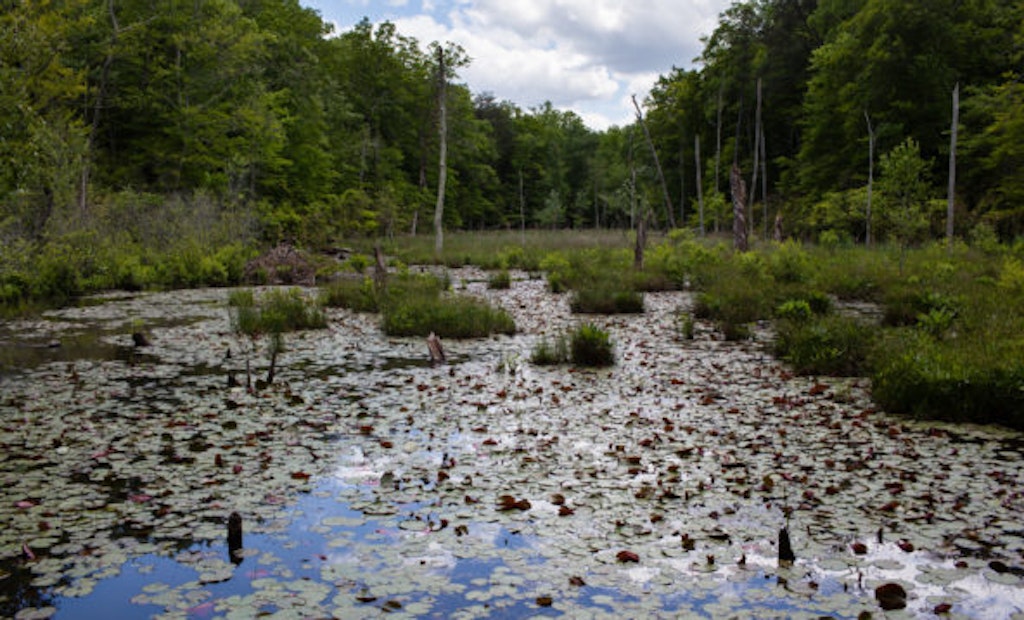
Wetlands at Calvert Cliffs State Park and Calvert County, Maryland. (Photo by Will Parson / Chesapeake Bay Program)
Chesapeake Conservancy’s data science team has developed an artificial intelligence deep learning model for mapping wetlands, which resulted in 94% accuracy. Supported by EPRI, an independent, non-profit energy research and development institute; Lincoln Electric System; and the...